The Emerging Role of Metabolomics and Proteomics in the Development of Cardiovascular Biomarkers
There are several unmet needs for cardiovascular disease (CVD) biomarkers. For example, we lack specific circulating biomarkers that provide biochemical proof of myocardial ischemia and very early myocardial infarction. Knowledge of new circulating markers of susceptibility to CVD may provide more precise estimates of risk, while also defining the pathways perturbed in individual patients, revealing new targets for intervention, and ultimately enabling an individualized approach to care.1, 2
To address unmet needs in disease biomarkers, investigators have turned to comprehensive profiling of large numbers of endogenous metabolites and proteins in biofluids, which has been termed “metabonomics”3 or “metabolomics” and “proteomics”4, respectively (Figure 1). There are approximately 20-25,000 genes. However, there are substantially more proteins generated (Figure 2), indicating that the proteome is constantly changing according to the interactions between the genome and the environment. “Metabolomics,” or metabolic profiling, details the metabolic intermediates as a result of protein enzymatic actions on the substrates. Metabolites and proteins are the end result of all the regulatory complexity present in a cell, tissue, or organism, including transcriptional and translational regulation as well as post-translational modifications. Therefore, metabolites and proteins are the most proximal reporters of the body’s response to a disease process or drug therapy and they constitute the molecular basis of personalized medicine (Figure 2). As described below, emerging metabolomics and proteomics techniques will allow us to “overlay” new biomarkers on existing cardiovascular disease biomarkers and multimarker scores.5 It is anticipated that some new markers will be uncorrelated or “orthogonal” to existing markers, thus providing additional pathobiological insights and information for cardiovascular disease management.6
Methods for Metabolic Profiling
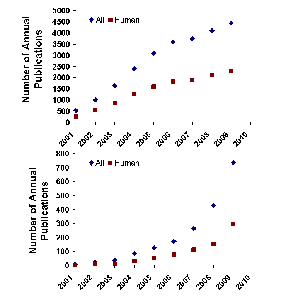
Two core technologies are utilized to perform metabolic profiling: nuclear magnetic resonance (NMR) and tandem mass spectrometry (MS/MS) (for reviews of metabolomics technologies, see articles by Dunn,9 Mayr,10 and Lindon11). NMR requires relatively little sample preparation and is nondestructive, allowing for subsequent structural analyses. However, the method tends to have low sensitivity and can detect only highly abundant analytes. MS/MS, coupled with liquid chromatography (LC), on the other hand, has much higher sensitivity for small molecules and is also applicable to a wide range of biological fluids (including serum, plasma, and urine). Recent advances in MS technology now enable researchers to determine analyte masses with such high precision and accuracy that metabolites can be identified unambiguously even in complex fluids.
These technologies can be used to characterize biological samples either in a targeted manner, or in a pattern discovery manner. In the former, the investigator targets a predefined set of metabolites for analysis. In the latter, the investigator is faced with a complex pattern of peaks, many of which are anonymous—the molecular identities of the species giving rise to the peaks are generally not known. While the targeted approach is more limited, the analysis is more straightforward as the biochemicals giving rise to the signals have already been identified. The pattern discovery or fingerprint approach is inherently less biased, but the need for subsequent unambiguous identification of the peaks can be difficult.
Statistical Approaches and Data Reduction for “Omics” Approaches
Although high-throughput metabolomics and proteomics approaches to biomarker discovery bring many advantages, they also bring the danger of generating false-positive associations due to multiple testing and overfitting of data. Replication is imperative to minimize overfitting of data.12 Application of traditional statistical approaches (e.g., Bonferroni correction) in this setting tends to levy an insurmountable statistical penalty that can obscure biologically relevant associations. Newer statistical techniques, such as advanced resampling methods or control of the false discovery rate,13 coupled with functional trend analysis in which changes in constituents of common pathways are analyzed together, can aid in detecting subtle but important changes in multiple variables identified in “omics” approaches.14-16 Several data reduction strategies can be used to construct multivariate metabolite biomarker profiles,17 including discriminant analysis,18 partial least squares, principal components analysis,19 and artificial neural networks.20Application of Metabolomics for Cardiovascular Biomarker Discovery
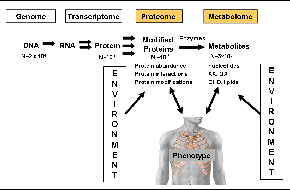
To help circumvent these problems, investigators have applied these emerging technologies to unique clinical scenarios where serial sampling can be performed in patients both before and after a controlled perturbation, thereby allowing each patient to serve as his or her own biological control.16, 23, 24 As proof of principle, our group has applied targeted MS-based metabolomics to patients undergoing “planned myocardial ischemia”16, 24 elicited by exercise and “planned myocardial infarction” induced by alcohol septal ablation in patients with hypertrophic obstructive cardiomyopathy.23 In both cases, robust metabolic changes were evident within minutes of the physiologic perturbation, when traditional markers of myocardial injury such as troponin remained unchanged. In planned myocardial infarction (PMI) circulating levels of metabolites participating in purine and pyrimidine metabolism, the tricarboxylic acid cycle and its upstream contributors, and the pentose phosphate pathway were discovered in an initial derivation group and were validated in a second, independent group of PMI patients and in patients with spontaneous myocardial infarction.
Metabolic profiling has also demonstrated distinct patterns of myocardial substrate utilization in individuals undergoing surgical coronary revascularization. Turer et al. found that patients with left ventricular dysfunction demonstrated global suppression of metabolic fuel uptake and limited myocardial metabolic reserve and flexibility after global ischemia/reperfusion stress in the setting of cardiac surgery.25 Shah and colleagues used a targeted MS/MS-based platform to profile 69 metabolites in over 600 subjects from the Duke CATHGEN biorepository who underwent cardiac catheterization to evaluate suspected ischemic heart disease.26 They demonstrated that peripheral blood metabolite profiles, one enriched for branched-chain amino acid (BCAA) metabolites and another comprising urea cycle metabolites, add to the discriminative capability for coronary artery disease compared with models containing only clinical variables.
Methods for Protein Profiling
Proteomics relies on many of the same principles and technologies as metabolomics (i.e liquid chromatography-mass spectrometry)28, 29 but offers a unique set of challenges. The proteome contains many more constituents than the metabolome (>Figure 2) and has an estimated dynamic range in human plasma greater than 1010, which makes surveying low-abundance proteins particularly challenging. To access proteins at lower abundance (e.g., less than 100 ng/L in plasma, concentrations at which many known protein biomarkers such as carcinoembryonic antigen, prostate-specific antigen, and the troponins occur), has historically required multidimensional protein fractionation and extensive LC-MS-MS analysis that can take weeks for a single case-control sample pair. Small sample sizes relative to the high dimensionality of the proteome and the scale of normal variation in the human population has resulted in frequent false positives arising from biological or technical variability and a paucity of proteomics-derived clinical biomarkers.However, recent technological advances in proteomics, fueled in part by NHLBI initiatives,30 hold great promise for advancing proteomics-based discovery of biomarkers. These advances include the PeptideAtlas for databasing and statistical validation of mass spectrometry data;31 ultra-high sensitivity MS platforms (i.e. cryogenic Fourier-transform MS,32 and accurate inclusion mass screening [AIMS] of peptides),33 advances in sample throughput (microfluidic chips;34 peptoid-based microarrays),35 and in quantitative measurement of protein candidates (i.e. stable isotope dilution–multiple reaction monitoring MS (SID-MRM-MS).36
With the aforementioned challenges in mind, candidate biomarkers being identified by discovery-based proteomics will require extensive qualification and validation.29 The verification process that bridges findings from discovery-based proteomics to quantitative assay configuration should ideally be high throughput, involve low sample volume, and not rely on antibodies to each candidate protein, because antibodies do not exist for many identified proteins. AIMS can provide rapid, semiquantitative qualification of approximately 100 proteins/week in patient blood. SID-MRM-MS can then be employed to measure signature or proteotypic tryptic peptides that uniquely and stoichiometrically represent the protein candidates of interest.
Application of Proteomics for Cardiovascular Biomarker Discovery
MRM-based assays for proteins for which antibody reagents are not available was recently reported for cardiovascular disease markers troponin and IL-33.37 Thus, MS is a promising technology to complement immunoassays for biomarker validation. In addition, profiling of samples from large patient cohorts, stratified by known risk factors or exposures, will reduce the impact of clinical confounding variables.19 Proteomics studies are beginning to be performed on larger cohorts; a recent urinary proteomics study associated 238 polypeptides with coronary artery disease in a derivation cohort (N=408) and then utilized the multi-marker protein pattern to distinguish subjects with and without CAD in a second blinded cohort (N=138) with a C-statistic of 0.87.38
Conclusion
Although metabolomics and proteomics technologies are still under development, they complement other functional genomic approaches, such as high throughput genome sequencing, and RNA expression analysis.39 Together, they hold great promise to transform our ability to profile samples and discover multiple new biomarkers for CVD. Novel multi-marker approaches may also permit identification of alterations in specific CVD pathways to inform the best preventative and therapeutic interventions for each individual, by restoring the specific perturbed pathways to their normal function.References
- Cortese DA. A vision of individualized medicine in the context of global health. Clin Pharmacol Ther. 2007;82(5):491-493.
- Braunwald E. The Simon Dack lecture. Cardiology: the past, the present, and the future. J Am Coll Cardiol. 2003;42(12):2031-2041.
- Nicholson JK, Lindon JC, Holmes E. 'Metabonomics': understanding the metabolic responses of living systems to pathophysiological stimuli via multivariate statistical analysis of biological NMR spectroscopic data. Xenobiotica. 1999;29(11):1181-1189.
- James P. Protein identification in the post-genome era: the rapid rise of proteomics. Q Rev Biophys. 1997;30(4):279-331.
- Zethelius B, Berglund L, Sundstrom J, et al. Use of multiple biomarkers to improve the prediction of death from cardiovascular causes. N Engl J Med. 2008;358(20):2107-2116.
- Sabatine MS, Morrow DA, de Lemos JA, et al. Multimarker approach to risk stratification in non-ST elevation acute coronary syndromes: simultaneous assessment of troponin I, C-reactive protein, and B-type natriuretic peptide. Circulation. 2002;105(15):1760-1763.
- Wishart DS, Tzur D, Knox C, Eisner R, Guo AC, Young N, Cheng D, Jewell K, Arndt D, Sawhney S, Fung C, Nikolai L, Lewis M, Coutouly MA, Forsythe I, Tang P, Shrivastava S, Jeroncic K, Stothard P, Amegbey G, Block D, Hau DD, Wagner J, Miniaci J, Clements M, Gebremedhin M, Guo N, Zhang Y, Duggan GE, Macinnis GD, Weljie AM, Dowlatabadi R, Bamforth F, Clive D, Greiner R, Li L, Marrie T, Sykes BD, Vogel HJ, Querengesser L. HMDB: the Human Metabolome Database. Nucleic Acids Res. 2007;35(Database issue):D521-526.
- Kyoto Encyclopedia of Genes and Genomes. Available at: http://www.genome.jp/kegg. Accessed September, 2010.
- Dunn WB, Bailey NJ, Johnson HE. Measuring the metabolome: current analytical technologies. Analyst. 2005;130(5):606-625.
- Mayr M. Metabolomics: ready for the prime time? Circ Cardiovasc Genet. 2008;1(1):58-65.
- Lindon JC, Holmes E, Nicholson JK. Metabonomics techniques and applications to pharmaceutical research & development. Pharm Res. 2006;23(6):1075-1088.
- Ransohoff DF. Rules of evidence for cancer molecular-marker discovery and validation. Nat Rev Cancer. 2004;4(4):309-314.
- van der Greef J, Stroobant P, van der Heijden R. The role of analytical sciences in medical systems biology. Curr Opin Chem Biol. 2004;8(5):559-565.
- Mootha VK, Lindgren CM, Eriksson KF, et al. PGC-1alpha-responsive genes involved in oxidative phosphorylation are coordinately downregulated in human diabetes. Nat Genet. 2003;34(3):267-273.
- Berriz GF, King OD, Bryant B, Sander C, Roth FP. Characterizing gene sets with FuncAssociate. Bioinformatics. 2003;19(18):2502-2504.
- Sabatine MS, Liu E, Morrow DA, et al. Metabolomic identification of novel biomarkers of myocardial ischemia. Circulation. 2005;112(25):3868-3875.
- Villas-Boas SG, Moxley JF, Akesson M, Stephanopoulos G, Nielsen J. High-throughput metabolic state analysis: the missing link in integrated functional genomics of yeasts. Biochem J. 2005;388(Pt 2):669-677.
- Manly M. Multivariable Statistical Methods: A Primer. 1994 ed. London: Chapman & Hall.
- Raamsdonk LM, Teusink B, Broadhurst D, et al. A functional genomics strategy that uses metabolome data to reveal the phenotype of silent mutations. Nat Biotechnol. 2001;19(1):45-50.
- Bishop C, ed. Neural Networks for Pattern Recognition. Oxford: Clarendon Press; 1995.
- Roe CR, Millington DS, Maltby DA. Identification of 3-methylglutarylcarnitine. A new diagnostic metabolite of 3-hydroxy-3-methylglutaryl-coenzyme A lyase deficiency. J Clin Invest. 1986;77(4):1391-1394.
- Kirschenlohr HL, Griffin JL, Clarke SC, et al. Proton NMR analysis of plasma is a weak predictor of coronary artery disease. Nat Med. 2006;12(6):705-710.
- Lewis GD, Wei R, Liu E, et al. Metabolite profiling of blood from individuals undergoing planned myocardial infarction reveals early markers of myocardial injury. J Clin Invest. 2008;118(10):3503-3512.
- Lewis GD, Farrell L, Wood MJ, et al. Metabolic signatures of exercise in human plasma. Sci Transl Med. 2(33):33ra37.
- Turer AT, Stevens RD, Bain JR, et al. Metabolomic profiling reveals distinct patterns of myocardial substrate use in humans with coronary artery disease or left ventricular dysfunction during surgical ischemia/reperfusion. Circulation. 2009;119(13):1736-1746.
- Shah SH, Bain JR, Muehlbauer MJ, et al. Association of a peripheral blood metabolic profile with coronary artery disease and risk of subsequent cardiovascular events. Circ Cardiovasc Genet. 3(2):207-214.
- Lewis GD, Gerszten RE. Toward metabolomic signatures of cardiovascular disease. Circ Cardiovasc Genet. 3 (2):119-121.
- Domon B, Aebersold R. Mass spectrometry and protein analysis. Science. 2006;312(5771):212-217.
- Rifai N, Gillette MA, Carr SA. Protein biomarker discovery and validation: the long and uncertain path to clinical utility. Nat Biotechnol. 2006;24(8):971-983.
- Ping P, Chan DW, Srinivas P. Advancing cardiovascular biology and medicine via proteomics: Opportunities and present challenges of cardiovascular proteomics. Circulation.121(21):2326-2328.
- Deutsch EW, Lam H, Aebersold R. PeptideAtlas: a resource for target selection for emerging targeted proteomics workflows. EMBO Rep. 2008;9(5):429-434.
- Lin C, Mathur R, Aizikov K, O'Connor PB. First signal on the cryogenic Fourier-transform ion cyclotron resonance mass spectrometer. J Am Soc Mass Spectrom. 2007;18(12):2090-2093.
- Jaffe JD, Keshishian H, Chang B, Addona TA, Gillette MA, Carr SA. Accurate inclusion mass screening: a bridge from unbiased discovery to targeted assay development for biomarker verification. Mol Cell Proteomics. 2008;7(10):1952-1962.
- Stanislaus R, Arthur JM, Rajagopalan B, Moerschell R, McGlothlen B, Almeida JS. An open-source representation for 2-DE-centric proteomics and support infrastructure for data storage and analysis. BMC Bioinformatics. 2008;9:4.
- Udugamasooriya DG, Dineen SP, Brekken RA, Kodadek T. A peptoid "antibody surrogate" that antagonizes VEGF receptor 2 activity. J Am Chem Soc. 2008;130(17):5744-5752.
- Keshishian H, Addona T, Burgess M, Kuhn E, Carr SA. Quantitative, multiplexed assays for low abundance proteins in plasma by targeted mass spectrometry and stable isotope dilution. Mol Cell Proteomics. 2007;6(12):2212-2229.
- Kuhn E, Addona T, Keshishian H, et al. Developing multiplexed assays for troponin I and interleukin-33 in plasma by peptide immunoaffinity enrichment and targeted mass spectrometry. Clin Chem. 2009;55(6):1108-1117.
- Delles C, Schiffer E, von Zur Muhlen C, et al. Urinary proteomic diagnosis of coronary artery disease: identification and clinical validation in 623 individuals. J Hypertens. 2010;28(11):2316-22.
- Shah SH, Hauser ER, Bain JR, et al High heritability of metabolomic profiles in families burdened with premature cardiovascular disease. Mol Syst Biol. 2009;5:258.
Keywords: Biomarkers
< Back to Listings