AI in Cardiology: Science vs. Science Fiction
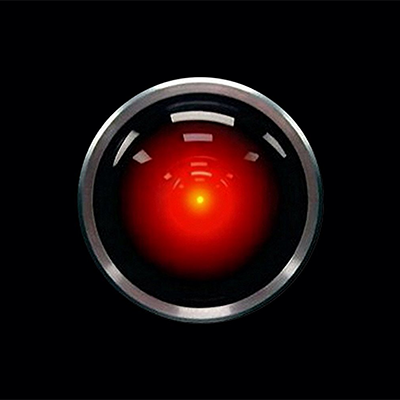
Dave: "Open the pod bay doors, Hal."
Hal: "I'm sorry, Dave, I'm afraid I can't do that."
… "This mission is too important for me to allow you to jeopardize it."
– 2001: A Space Odyssey
In a subplot of the 1968 classic 2001: A Space Odyssey, an astronaut (Dave) narrowly escapes death when his autopiloting system (Hal) attempts to rout deactivation. Prompting discussion on the limitations and dangers of technology, this film raises fears emblematic of the concerns surrounding the use of artificial intelligence (AI) in health care: (1) Can AI help physicians make better clinical decisions? (2) Will AI inevitably replace human judgement?
History
Early efforts in AI focused on clear and logical algorithms. Computers had limited processing capacity and were incapable of learning. Yet within a few years of 2001: A Space Odyssey's debut, a Stanford physician and computer scientist Edward Shortliffe, developed an if-then heuristic program for diagnostic and therapeutic assistance in the identification and management of infectious disease. While the implementation of this system was far from perfect, it consistently outperformed the experts.
Decades later, a renaissance in AI has occurred with technological advances in computing power and the invention of deep learning neural networks, allowing for the mixture of logic and probabilities. Deep learning is a class of algorithms using multiple layers to extract higher-level features from raw input. Each intermediate layer contains mathematical equations and weights that influence the probability of a given outcome. Learning occurs iteratively as these weights are adjusted to improve the accuracy of the output. Current applications include:
- Natural language processing (NLP): voice dictation, medical literature and electronic health records (EHR) searches.
- Medical image analysis: diagnostics, computer vision and robotics.
- "Big data" analytic methods: gene expression, proteomics and metabolomics.
In fact, science fiction predicts AI systems will eventually construct models themselves, discover logical rules, and understand the context and the consequences of their actions. Lore insists that these machines will be capable of self-improvement and self-perpetuation. If this narrative is to be believed, AI will take over most of our routine work.
Limitations
Real-world examples of AI success and failure abound. In the past decade, IBM's medical division set out to develop their supercomputer Watson as a tool for redefining patient care. After achieving some success with their cognitive radiology assistant, AI software that identifies imaging abnormalities and searches the EHR for corroborating information, their clinical diagnosis and management software failed to reliably integrate patient symptoms and other data sources into a coherent clinical approach: "If the doctor input that the patient was suffering from chest pain, the system didn't even include heart attack, angina pectoris or a torn aorta in its list of most likely diagnoses. Instead, Watson thought a rare infectious disease might be behind the symptoms." IBM blamed their early failures on insufficient data in their training sets.
In fact, it has been widely postulated that "deconvoluting the information content embedded in functional genomics requires a substantial change in the scale of modern clinical assessment." The clinical history and physical exam need to become more structured, detailed and universal. With the proliferation of wearable and portable technologies – and the internet-of-things – the seamless integration of data sources and databases promises to facilitate future data collection, storage and analysis.
While digital morphometry and wearable technologies generate voluminous data, it is unclear how effectively this data directly impacts patient care. Consider the Apple Heart Study conducted by Stanford: 419,297 participants used the Apple watch to identify arrhythmia. Atrial fibrillation was detected in 2161 (0.52%) participants; however, only 775 were 65 years or older and 25.5% of 2,161 asymptomatic individuals had a duration longer than 24 hours, suggesting the study intervention only changed management in about 200 individuals (less than 0.05%).
Although popular culture has proclaimed AI will make society equitable, the algorithms propel the biases of the underlying data into the output. Recall the Google algorithm that labeled an Afro-Caribbean couple as gorillas. Amazon stopped using AI to filter new job candidates after realizing the data created from their pool of predominately male employees trained the system to exclude women.
While AI and robotics have come a long way, these systems remain far inferior to human beings in terms of emotional intelligence, abstraction, creativity and discovery. Consequently:
- Nearly all language processing software fails to correctly interpret complex sentence structure, phrases like "could not be ruled out," which are part of routine medical jargon.
- Lacking sufficiently human-like sensors, computers remain poor at interactive physical tasks.
- For large and complex data sets, the iterative approach employed by most AI systems weakens statistical inferences through multiple comparisons, resulting in overfitted data and false conclusions.
Ethics
The integration of AI into health care continues to raise important ethical issues. Concerns range from the adequacy of informed consent to the monetisation of patient data. For instance:
- Consent and privacy: Consider the potential consequences of the release of ultra-detailed personal health information. Would insurance rates rise for individuals with adverse genetics markers, dietary habits or environmental exposures? Would employers limit job prospects due to a predicted propensity toward certain diseases?
- Observation of at-risk-populations: Investigators need a pre-existing plan and timeline for population surveillance and intervention. Recall 1932 Macon County, Alabama, where the U.S. Public Health Service initiated a "natural-history" study of syphilis in 400 African American men. Arsenic and bismuth compounds used for treatment of the disease at the time of study initiation were not offered to the research subjects, and when highly effective therapy with penicillin became available in the 1940s and 1950s, this drug was also withheld. Originally designed to last for six to eight months, this study continued for 40 years.
- Social messaging: Stakeholders sway public opinion. Consider the dubious claims of the direct-to-consumer genetic testing companies ranging from personal health counselling to assistance finding the perfect mate. Timothy Caulfield, a University of Alberta Professor of Law and Public Health, pointed out this industry ignores the 99.9% of DNA identical in every person, and instead reifies the social construct of race, perpetuating "scientifically inaccurate and socially harmful ideas about difference."
- Financial incentives: Finally, the creation of new systems for personalized data collection, storage and analysis will require investment in infrastructure, analytics and security. Who owns this information: the patient or the institution? Consider the 2015 scandal when Cambridge Analytica acquired private data from more than 50 million Facebook users to build psychological profiles of the American voter. Will patients' information be similarly exploited by collecting companies looking for business opportunities?
Potential Solutions
In 2018, the Montréal Declaration of Responsible AI was released. Agreed upon by more than 100 different technology and ethics experts, this document is a guide for individuals, engineers, researchers, and business professionals in the responsible and ethical use AI technology. It proposes shared responsibility and benefit from the collection and analysis of personal data, emphasizing a robust process of informed consent and an ability to withdrawal data from analysis at any time.
Final Thoughts
AI has been under development for decades. A convergence of new technologies, big data, and innovation is driving a new wave of algorithmic and probabilistic models with the goal of earlier disease detection and intervention. New techniques in data science still require an understanding of the underlying medicine and experimental design. Cardiology has a long history of successfully integrating basic and translational science. So, take heart my fellow trainees, Hal cannot push us out of the air-lock just yet...