For the FITs | The Evolving Roles of Digital Health, Big Data and AI: "Tech-celeration" in CV Care
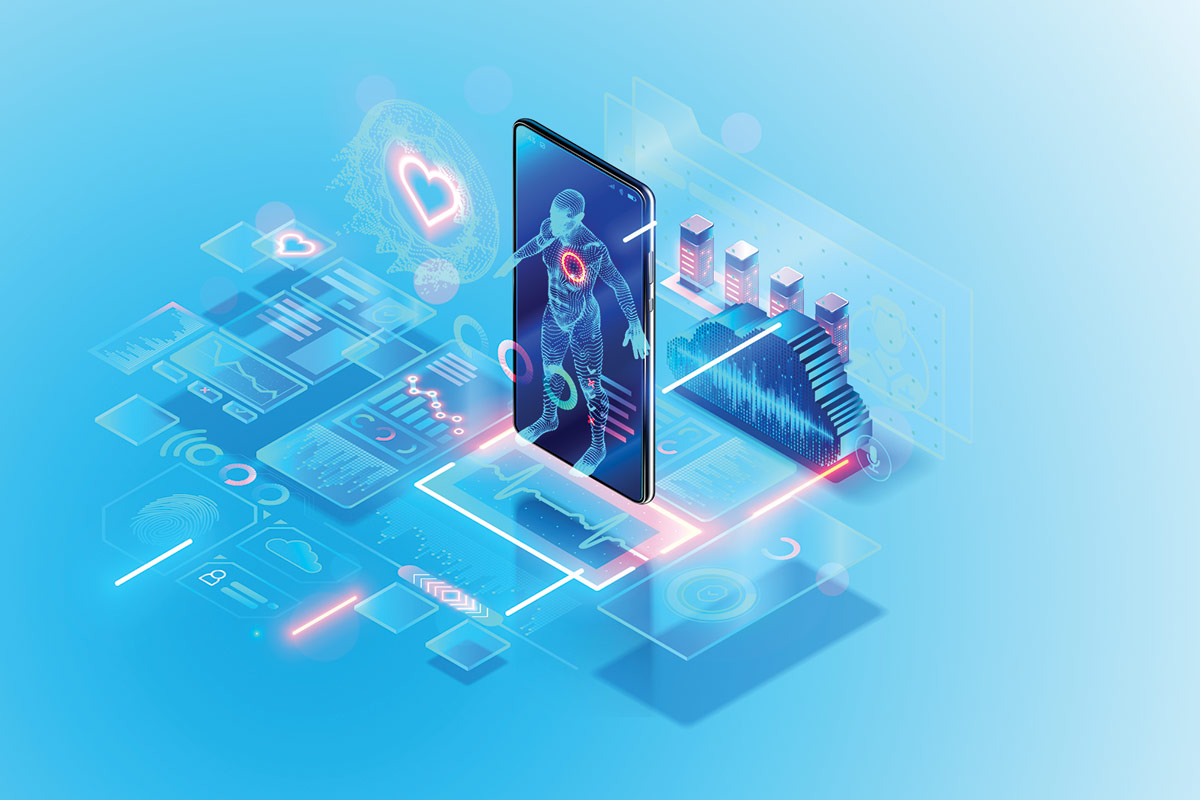
On a clinic day in spring 2021, I logged into a virtual clinic visit with Mr. B. He often missed clinic visits because he lacked reliable transportation, and forgot to bring his pill bottles and couldn't recall the medications he took. Now Mr. B. was temporarily living in North Carolina and he'd traveled 500 miles back to New York City for his follow-up visit – all without leaving his daughter's kitchen table. He showed me his medicine bottles, the corner of the living room serving as the family's home gym and a smart scale his grandson had recently bought for him. He proudly reported he'd lost three pounds and showed me that his socks no longer left deep indentations on his shins.
Digital health is changing the way we practice and innovate in cardiology. As many of us have experienced, the COVID-19 pandemic catalyzed the fast adoption of technology-enhanced services so we could efficiently and safely care for patients. However, telehealth is just one component of the evolving ecosystem of digital health.
Framework of Digital Health
Digital health spans how technology is used to monitor health, prevent or diagnose disease, and deliver patient-centered care. It ranges from hardware (smartphones, wearables, sensors) to services (telehealth, remote monitoring, mHealth apps) to data management and interpretation (data science, electronic health records, artificial intelligence [AI]).
The five "technology categories" proposed in the Stanford Center for Digital Health Report published last year provides a useful framework.1 These technology buckets are not independent of each another. When cohesively used together, they can redefine how patients interact with the health care system. These categories are:
Wearables, Sensors and Other Biometric Monitoring Technologies (BioMeTs)
BioMeTs comprise sensor technologies that collect and process data. Often connected to a phone or network, they allow for real-time monitoring. BioMeTs span across direct-to-consumer products, including smartwatches such as the Apple watch, as well as prescribed products, like rhythm monitors including the Zio patch.2,3
Mobile and Web Applications
This cohort often serves as an interface between the user and the rest of the digital health technologies. Examples include apps that collect and organize data from BioMeTs and provide personalized coaching, such as Omada Health. The evolving arena of digital therapeutics, which are evidence-based and regulatory-adherent digital interventions, is a type of digital health product that often at least partially falls in this category.
Health IT, Infrastructure, Data Management
This information technology (IT) subgroup includes electronic health records and large repositories of data in the form of registries or data warehouses. Understanding what we can learn about cardiology from "big data" has been a recent area of interest for journals such as The Lancet.4 Companies such as Verily have partnered with academia to recruit patients into large data-collection initiatives (Project Baseline) to better understand health and transition to disease.
AI, Machine Learning and Algorithms
AI is a field of computer science focused on building machines capable of performing tasks that previously required human intelligence. Machine learning (ML) is a subset of AI where computer algorithms learn without explicit programming, and ML can be subcategorized into supervised and unsupervised learning. Recent work in AI as it applies to cardiology has focused on data extraction, diagnosis and classification of cardiac disease, and outcome prediction models to help guide cardiac management. As AI research continues to grow, there have been efforts to build consensus around ensuring high quality evidence generation and transparency in clinical trials evaluating interventions involving AI.5,6
New Clinical Care Models, Decentralized Trials
Recent innovations in this category include everything from virtual clinic visits to augmented reality cardiac rehab. While telehealth has been around for some time, we've seen a boom in adoption across all specialties. At least some portion of virtual visits has remained a part of outpatient care as a new normal. This has allowed for greater access to health care services for many patients, including those who live far from their care center, have time constrains due to jobs or caregiving duties, or lack transportation. Within clinical trials, there has been a move towards decentralized trials as a way to make participation in trials easier for patients and to improve recruitment and retention.
Where are We Now?
After the initial spike of digital health solutions for health care delivery during the Covid-19 pandemic, telehealth utilization has stabilized to about 13% to 17% of outpatient visits across all specialties.7 It's estimated that 232 million wrist-worn wearables will be shipped in 2021, and this number is expected to reach 380 million in 2025.8
The possibility for the ways digital health can enhance cardiac clinical care is exciting, especially as it pertains to diagnosis, patient monitoring and better understanding of our patients' health risk. At-home blood pressure cuffs have been used for many years to diagnose and monitor hypertension. This type of remote monitoring is being expanded to support our patients with other conditions such as heart failure, atrial fibrillation and coronary artery disease.9
Furthermore, digital health may provide an opportunity to better understand patient risk of cardiovascular complications. The vast majority of current risk calculators assess risk based on data at a specific point in time, without considering trends over time, a patient's baseline data, or data generated outside the health care system. BioMeTs provide an opportunity to enhance risk assessment and ML holds the potential to screen the entirety of a patient's data faster and more accurately. Another exciting change has been the evolution of decentralized clinical trials, which can include virtual recruitment, virtual visits, mobile or local health care providers, and even remote data gathering that does not require patients to come into the clinic.10
However, continued growth of digital health and subsequent positive impact on patient health is not inevitable. While there's a lot of potential, there are also important limitations and challenges to solve. A current area of focus surrounds challenges involved in the adoption of digital health technologies in clinical practice, including confirming data accuracy, ensuring patient privacy, promoting data integration, and separating the generated information that actually adds diagnostic value from the noise.9
There are also the larger concerns of how to integrate these technologies into current health care delivery practices, and how these delivery practices should evolve given our increased ability to connect with patients via technology. Addressing these concerns requires multidisciplinary teams, more shared language in the field, and consensus around verification and validation of digital health technologies.11 Addressing these challenges are a focus of the next wave of evolution in digital health.
How Can You Get Involved?
Applying AI in HF
AI has a long and fascinating history. Yet it's the rise of graphic processing units (GPUs), which are capable of parallel processing that led to greater magnitudes in the growth of computing speed, and the internet and ImageNet, which brought millions of figures of content together to allow development of algorithms, that spurred the fast growth in AI and ML since the early 2010s.
In cardiology, the three main AI categories are data extraction, diagnosis and classification of cardiac disease, and outcome prediction models to guide cardiac management. Here's how these have been used in heart failure (HF).
Data extraction: Development of accurate diagnostic and prognostic tools depends on accurate inputs. Leveraging AI for data acquisition from images and clinical notes is a large area of focus. This has quickly expanded from ECG data to using various imaging data as inputs along with natural language processing to glean information from clinical notes.13,14
Diagnosis and classification of cardiac disease: ML has been used to identify a HF diagnosis and classify types of HF failure in the outpatient and inpatient settings.15-20 Data used include clinical, laboratory, ECG and echocardiographic inputs.
Outcome prediction models: One of the first papers on this topic was published in 1995 and showed that a neural network could use echocardiography data to predict one-year mortality in 95 patients with HF.21 The accuracy of this model was superior to a linear model or clinical judgment. Since then, a number of additional studies have shown use cases for ML to predict hospitalization, readmission or death in patients with HF.22
Health data is being redefined and digital health is providing unprecedented access to monitor patients longitudinally. Many fellows wonder how they can get involved in this growing field. I believe some of the best advances happens when we let our curiosity be the guide. Here are some options on how to jump in, questions I've been pondering and possible next steps:
Clinician or Clinical Leader
As digital health becomes an integral part of connecting with and treating our patients, it's important to reflect on the current patient experience and consider what it should be. How do we redesign the health care system to truly be patient centered? How do we streamline integration and promote adoption of validated technologies into our health care systems? What digital health tool might help me take care of the patient in front of me? How do we keep our clinical community updated on the latest, evidence-based innovations?
Further training isn't needed to ask and answer these questions. However, if leading organizations and driving organizational change is of interest, consider pursuing an MBA for leadership and management training. Also, expand your knowledge base by reading digital health journals, such as The Lancet Digital Health.
Entrepreneur or Advisor
Guiding teams towards solving clinically relevant questions is the most important value a physician can bring to digital health innovation. Your clinical knowledge, honed over years of training, can help guide the development of useful digital health tools. Some questions to ponder: How can we combine principles of behavioral economics into digital health to affect patient behavior and engagement in their health? What clinical questions lend themselves to better answers if we knew more about our patients? How can we use digital health and AI to combat racial and other disparities instead of propagating them?12
Coursera courses are a good starting point for learning about designing and innovating in digital health and AI. For ML, consider courses by Andrew Ng or Matthew Lungren. For more formal IT training, consider a fellowship or masters in clinical informatics.
The journey into digital health often starts close to home. Does your institution have a digital health center or a transformation, innovation or tech transfer office? Reach out to get involved and for introductions to entrepreneurs and others working in this space. And consider joining a local chapter of the Society of Physician Entrepreneurs.
Researcher or Thought Leader
Just as medicines and devices are rigorously tested for efficacy and safety, we need to build a field of evidence generation for digital health initiatives and AI algorithms. How do we prove the effectiveness of these digital interventions? What do "strategy trials" in digital health look like?
Other research questions include: What data can we generate remotely that may help augment our understanding of patient risk? What do these updated risk scores or models look like? How do we test these tools and successfully implement digital health technologies into clinical practice? How do we ensure patient safety and security of patient data? What data should we use to build the "ImageNet" for cardiology and medicine more generally? Looking even farther into the future, what does a decentralized internet mean for a patient's ability to truly own their own data, and how will that impact clinical care and clinical research?
Asking and answering these kinds of questions may lead to roles within professional societies like the ACC, government organizations, industry or health care system leadership. To build your research in this field, it's important to find mentors who can help guide the launch of your research career. Start by looking within your institution for a digital health center. Attend national conferences such as the American Medical Informatics Association Annual Symposium and consider joining professional societies such as The Digital Medicine Society to learn about ongoing initiatives in the field.
The bond between technology and clinical medicine is only getting stronger. Medicine must evolve to meet patients where they are. Investing in and advancing digital health and AI innovation in medicine is important to improve what matters most: high quality and high value care at the (possibly virtual) bedside.
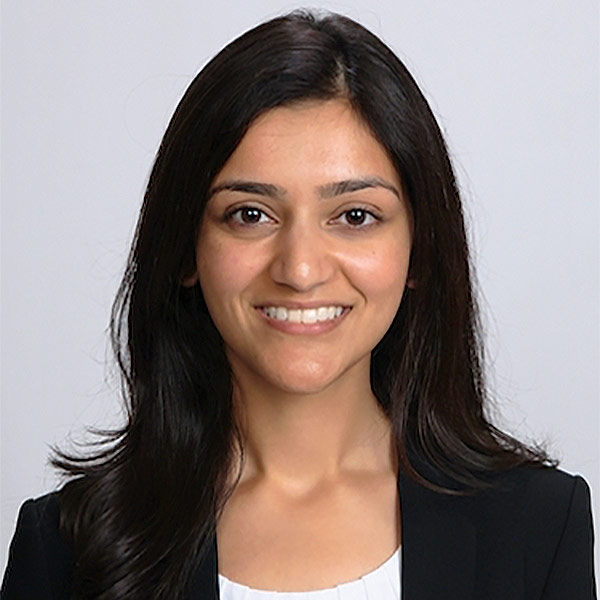
This article was authored by Sneha S. Jain, MD, MBA, a general cardiology fellow at Stanford Health Care in Palo Alto, CA. She graduated from the Johns Hopkins School of Medicine and Harvard Business School and completed her internal medicine residency at Columbia University Irving Medical Center in New York City. Jain acknowledges Ankit S. Jain, Andrea R. Coravos, and Ersilia M. DeFilippis, MD, for their insights and feedback on this article.
References
- Seninger C, Mainz M, Gupta A, et al. Stanford center for digital health landscape report. Stanford, 2020.
- Perez MV, Mahaffey KW, Hedlin H, et al. Large-scale assessment of a smartwatch to identify atrial fibrillation. N Engl J Med 2019;381:1909-17.
- Manta C, Jain SS, Coravos A, et al. An evaluation of biometric monitoring technologies for vital signs in the era of COVID-19. Clin Transl Sci 2020;13:1034-44.
- Spencer S, Sarkar R. Cardiology and big data: a call for papers. Lancet 2021;397:1430.
- Cruz Rivera S, Liu X, Chan AW, et al. Guidelines for clinical trial protocols for interventions involving artificial intelligence: the SPIRIT-AI extension. Nat Med 2020;26:1351-63.
- Liu X, Rivera SC, Moher D, et al. Reporting guidelines for clinical trial reports for interventions involving artificial intelligence: the CONSORT-AI Extension. BMJ 2020;370:m3164.
- Bestsennyy O, Gilbert G, Harris A, Rost J. Telehealth: a quarter-trillion-dollar post-COVID-19 reality. McKinsey and Company 2020;29.
- Good Times for the Smart Wearables Market. CCS Insight. Available here.
- Bayoumy K, Gaber M, Elshafeey A, et al. Smart wearable devices in cardiovascular care: where we are and how to move forward. Nat Rev Cardiol 2021;18:581-99.
- Dash R. Decentralized trial in atrial fibrillation patients (DeTAP). Clinicaltrials.gov.
- Goldsack JC, Coravos A, Bakker JP, et al. Verification, analytical validation, and clinical validation (V3): the foundation of determining fit-for-purpose for Biometric Monitoring Technologies (BioMeTs). NPJ Digit Med 2020;3:55.
- Obermeyer Z, Powers B, Vogeli C, Mullainathan S. Dissecting racial bias in an algorithm used to manage the health of populations. Science 2019;366:447-53.
- Bazoukis G, Stavrakis S, Zhou J, et al. Machine learning versus conventional clinical methods in guiding management of heart failure patients—a systematic review. Heart Failure Reviews 2021;26:23-34.
- Zhang R, Ma S, Shanahan L, et al. Discovering and identifying New York heart association classification from electronic health records. BMC Med Inform Decis Mak 2018;18:48.
- Panahiazar M, Taslimitehrani V, Pereira NL, Pathak J. Using EHRs for heart failure therapy recommendation using multidimensional patient similarity analytics. Stud Health Technol Inform 2015;210:369-73.
- Blecker S, Katz SD, Horwitz LI, et al. Comparison of approaches for heart failure case identification from electronic health record data. JAMA Cardiol 2016;1:1014-20.
- Alonso-Betanzos A, Bolon-Canedo V, Heyndrickx GR, Kerkhof PL. Exploring guidelines for classification of major heart failure subtypes by using machine learning. Clin Med Insights Cardiol 2015;9:57-71.
- Austin PC, Tu JV, Ho JE, et al. Using methods from the data-mining and machine-learning literature for disease classification and prediction: a case study examining classification of heart failure subtypes. Journal Clin Epidemiol 2013;66:398-407.
- Isler Y. Discrimination of systolic and diastolic dysfunctions using multi-layer perceptron in heart rate variability analysis. Computers Biology Med 2016;76:113-9.
- Shah SJ, Katz DH, Selvaraj S, et al. Phenomapping for novel classification of heart failure with preserved ejection fraction. Circulation 2015;131:269-79.
- Ortiz J, Ghefter CG, Silva CE, Sabbatini RM. One-year mortality prognosis in heart failure: a neural network approach based on echocardiographic data. J Am Coll Cardiol 1995;26:1586-93.
- Jing L, Ulloa Cerna AE, Good CW, et al. A machine learning approach to management of heart failure populations. JACC: Heart Failure 2020;8:578-87.
Clinical Topics: Arrhythmias and Clinical EP, Cardiovascular Care Team, COVID-19 Hub, Diabetes and Cardiometabolic Disease, Heart Failure and Cardiomyopathies, Noninvasive Imaging, Prevention, Atherosclerotic Disease (CAD/PAD), Atrial Fibrillation/Supraventricular Arrhythmias, Acute Heart Failure, Echocardiography/Ultrasound, Exercise, Hypertension
Keywords: ACC Publications, Cardiology Magazine, Ambulatory Care, Artificial Intelligence, Atrial Fibrillation, Big Data, Blood Pressure, Cardiology, Cimetidine, Clinical Medicine, Consensus, Coronary Artery Disease, COVID-19, Data Accuracy, Data Management, Data Science, Data Warehousing, Delivery of Health Care, Echocardiography, Economics, Behavioral, Ecosystem, Electrocardiography, Electronics, Exercise, Exploratory Behavior, Family Characteristics, Feedback, Fellowships and Scholarships, Follow-Up Studies, Government, Health Personnel, Heart Diseases, Heart Failure, Hypertension, Information Technology, Inpatients, Intelligence, Internet, Internship and Residency, Judgment, Knowledge Bases, Laboratories, Leadership, Linear Models, Machine Learning, Medical Informatics, Mentoring, Mentors, Monitoring, Physiologic, Natural Language Processing, Neural Networks, Computer, New York City, North Carolina, Nuclear Family, Organizational Innovation, Outpatients, Patient Care Team, Patient Readmission, Patient Safety, Patient-Centered Care, Physicians, Privacy, Prognosis, Reading, Registries, Risk Assessment, Risk Factors, Smartphone, Technology, Telemedicine, Universities, Unsupervised Machine Learning, Wearable Electronic Devices
< Back to Listings