Innovating Health Care | Addressing Bias and AI
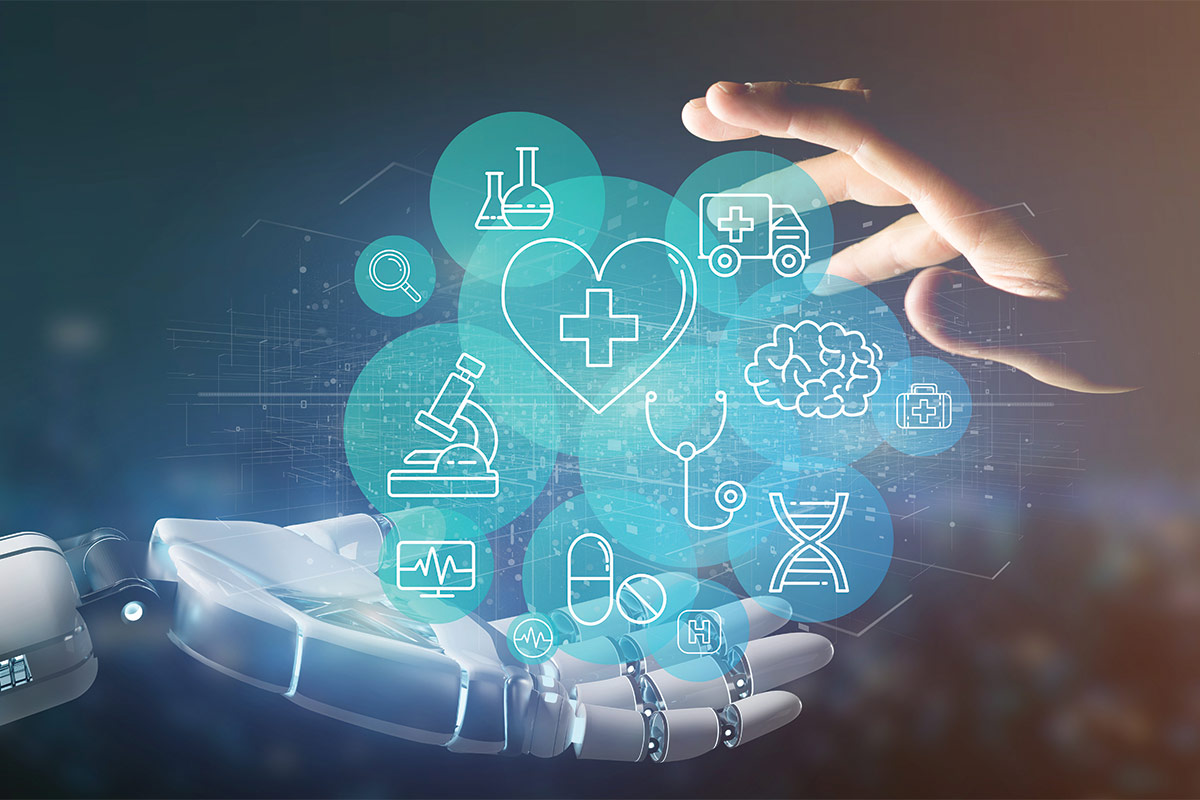
One of the most pressing issues in the conversation regarding artificial intelligence (AI) and machine learning in health care is how to address bias in designing AI algorithms. As the AI landscape evolves, many stakeholders, including clinicians, tech companies, investors, health care organizations and payers, are increasingly developing mitigation approaches. Sharing and evaluating these practices in an iterative mechanism will be essential to addressing algorithmic bias.
Best Practices in Addressing Bias
First and foremost, establishing governance frameworks for maintaining and using algorithms is among the most important practices for health care organizations looking to implement responsible AI. Such frameworks may include peer review, ethical forums, restricting use to only interpretable and explainable models, implementing methods to 'inspect' machine learning models, and checking model output to ensure it makes clinical sense.
Broader representation in the design, development, deployment and governance of AI is also needed. The underrepresentation of women and people of color in technology, and the under-sampling of these groups in the data shaping AI, has led to the creation of technology that is optimized for a small portion of the world.
Additionally, assessing fairness is essential in the data standardization that is the foundation of AI. As patterns emerge, they must be investigated and understood, rather than judged and dismissed. The focus now must be how to democratize data, review and reflect for bias and create systems that are applied globally and transcend social determinants and local inefficiencies in the health care delivery system.
The Role of Clinicians and Other Stakeholders
Clinicians must play an essential role in ensuring responsible use of AI by standardizing data, reviewing for bias, and taking clinical concepts and workflows and incorporating them into model learning procedures. Rather than training a system on data alone, incorporation of clinical points that are known to be physiologically important in a specific assessment modality can improve machine learning algorithm performance and engender clinician and patient trust.
Review of data is also essential to ensure that systems are taught to unlearn when a systemic algorithmic error or bias is recognized. Just as clinicians acquire data, integrate it and reassess their clinical management, the AI system must also be consistently reassessed and adjusted when needed.
Collaboration across silos is essential as well. Improving health and outcomes cannot be done without technology and innovations that industry can offer. Vendors also have a role in both ensuring good technology and offering transparent systems, as do medical societies like the ACC. Despite the massive capabilities of technology, the intrinsic power of AI is in identifying productive use cases and it is incumbent on medical societies to guide the application of these technologies to improve rather than impede clinical workflow and the clinician-patient experience.
At the end of the day, AI will not replace humans. However, the widespread application of AI technologies will assist in differentially focusing machine learning and human clinical acumen on appropriate unique tasks – all in support of higher quality care. It's then that the health care community can truly reap the benefits of the exponential growth in scientific knowledge while encouraging the human connection between patients and clinicians that is the art of diagnosis and healing.

Clinical Topics: Cardiovascular Care Team
Keywords: ACC Publications, Cardiology Magazine, Workflow, Artificial Intelligence, Skin Pigmentation, Social Determinants of Health, Trust, Machine Learning, Algorithms, Technology, Delivery of Health Care, Peer Review, Patient Outcome Assessment, Societies, Medical, Reference Standards, Innovation
< Back to Listings